Ensure The Success Of Your MMM (Market Mix Modeling) Project With This Standardized Process, By Mass Analytics’ Dr. Ramla Jarrar
In the first article we introduced our readers to the components of Marketing Mix Modeling, its role, and the environment in which it functions. In this article, we dive into the process that ensures the success of MMM projects.
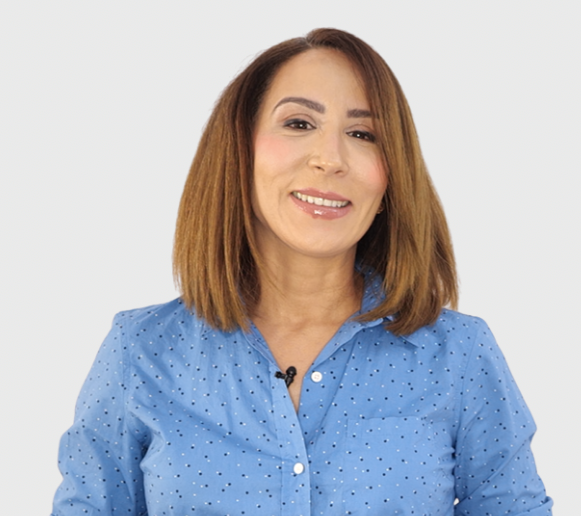
A reliable, replicable, and scalable process creates a sense of comfort regarding the success of a Marketing Mix Modeling (MMM) project. To achieve this, a standardized and well-defined workflow called CRISP-DM, is adopted, and adjusted to fit the nature of MMM projects. This type of workflow will not only guarantee a sense of comfort, but it will also make it effortless to update the project. In this article, we explore the CRISP-DM methodology and illustrate how we apply it to MMM projects.
The Six Steps of CRISP-DM:
The CRISP-DM Methodology -The CRoss Industry Standard Process for Data Mining- is a standard process of data science, and it is composed of six modules:
Business Understanding:
- Identifying the business questions that the analyst needs to answer throughout the project.
Data understanding:
- Deciding about the data that need to be prepared to address the business questions.
Data preparation:
- Collecting the data as it gradually comes in, and simultaneously preparing it.
Modeling:
- Defining the technique that will be deployed to answer the business questions
🡺 The constructed models must make sense commercially and statistically.
Evaluation:
- The evaluation of the recommendations and insights that are provided.
Deployment:
- Ensuring that the results, insights, recommendations, and all the outcomes of the data science project are going to be used by the company.
How CRISP-DM is used in the context of MMM:

In the context of Marketing Mix Modeling, the CRISP-DM consists of 5 steps that start by the project Kick-off and end with Results & Recommendations.
- The kickoff:
This step is similar to the business understanding module. Here, the main stakeholders of the project, discuss the general and specific objectives as well as deliverables of the project. Another objective of this step is to identify who the data owners are and who the project champion is. The latter will be the main project owner/contact and ensure that the MMM project achieves its goals.
- Generally speaking, a meeting is scheduled specifically for this phase.
- Data Collection and Understanding:
Usually, this starts by sending a data request from either the internal teams or the agency. This request will provide details about the data sets.
At this stage, the project champion ensures that the team have the right setup, internally, to start receiving and checking the data. That data must be checked continuously because it will be delivered in drips.
- Data Processing:
In simple terms, Data Processing is the step of the process where data starts telling a story. When data is received in its raw format, it never reflects how customers react to the different marketing activities. For example, when someone sees a TV Ad, it does not mean that they will immediately go to the store and buy the product. Typically, there is a type of delay between the moment the person is exposed to the ad, and the moment they decide to react to it. This is commonly called the carry-over effect. To model it, the analyst applies Ad Stock to the media variables. And to perfectly mimic how the customers respond to the different marketing activities, the analyst needs to apply other transformations to all the modeled variables.
Tip: Whether you are running the project as an agency, or internally within the client side, it is particularly important to make sure that you sit down again with the rest of the stakeholders and chart back all the data that you have received and processed. The aim is to be certain that the data is the right one. This would also be the right time to discover any data anomalies or to respond to any new questions.
- Model Building:
At this step, the analyst tries combinations of different variables to make sure that they properly model the data, or the KPI (Key Performance Indicator), that is added to the model.
The journey between data processing and modeling is an iterative one. In that, the analyst:
- Creates additional data
- Tests them in the model
- Consults the model and the residuals
- Collects extra variables and improves the fit as they go.
- Results and recommendations:
Before delivering the results and recommendations to the client, a pre-debrief meeting is highly recommended. The attendees of this meeting should be the project champion and those who are working closely on the project. Here, the initial results are communicated, and the attendees are used as a sounding board to see whether the results make sense to them or not.
Tip: In the final debrief meeting, when the results are presented to a room full of experts, it is advisable to have some support of someone who was at the pre-debrief meeting.
This step of the MMM workflow is the equivalent of the Deployment phase in CRISP-DM. This means the job of the analyst is not over once they create the results’ deck and disseminate the insights and recommendations. They should ensure that the following is implemented:
Deliver any needed follow-up.
If necessary, they debrief other entities, whether internally, or the agency with whom the client is collaborating.
The illustrated standard marketing mix process, which is based on CRISP-DM, is followed by every single agency and client. It is reliable and it allows the business to scale the way they see appropriate. It eventually leads to everyone involved in the project to embrace the results and the recommendations that have been delivered to the business.
In the following article we tackle the question of the data required for Marketing Mix Modeling project to get off the ground. Stay tuned!