Data Exploration: Uncover the Story Behind Each Variable, By MASS Analytics’ Dr. Ramla Jarrar
This is the fourth installment in our series covering the end-to-end Marketing Mix Modeling (MMM) process. Previously, we looked at the different data requirements for an MMM project. The next step is data exploration, where the analyst begins to understand the data and to shape their thinking for the work ahead.
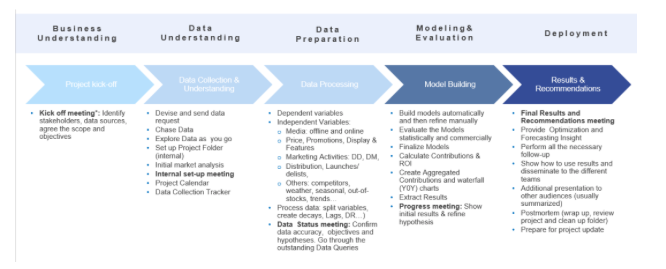
Now that we have the data for our marketing mix modeling project, the next step is making sense of it. The high volume of data can be overwhelming, but by following a simple step-by-step process, every variable will start falling into place. This is where data exploration comes in!
Data exploration is essential in marketing mix modeling because it allows you to understand the data you’re working with. Done right, you will be able to confidently proceed to the next phase, data processing. You’ll have a good idea of what transformations you need to apply on the variables.
When exploring your data, you need to be thorough in your analysis with each variable. You need to carefully use the statistical tools at your disposal. The following is a list of the main tools we recommend:
Chart! Chart! Chart!
Charting is by far the most important task at this step and should be conducted for each variable. If possible, charting the data by period, cross-section or region would reveal additional insights.
You can also chart independent variables, like the ones for media and marketing, against their modeled KPIs, for instance, sales. This can reveal any correlations that are influencing the modeled variables.
Univariate Analysis
We also recommend conducting univariate analysis for each of the variables to better understand their distribution. Examples of this are calculating:
- The mean
- Median
- Standard deviation
- Quartiles
The Correlation Matrix
With a correlation matrix, you can measure the linear relationship between two variables. Additionally, you can use a correlation function to study the lagged effect of a variable on their KPI. This is particularly important in marketing mix modeling since promotions and advertising generally take time to reveal their impact on sales. So, by delaying the independent variable, you can potentially see the correlation it has with the modeled KPI.
Scatterplots
Scatterplots allow you to visualize the trend of a variable compared against another. It’s another good way to see the strength of correlation between the variables you are analyzing.
And there you have it! By applying these techniques, you’ll be setting yourself for success in the next step of your marketing mix modeling project, which is data processing! This is where you transform your variables so that they start to make sense to your business. More on that in the next article!